LARA: Attribute-to-feature Adversarial Learning
for New-item Recommendation
ABSTRACT
Recommending new items in real-world e-commerce portals is a challenging problem as the cold start phenomenon, i.e., lacks of user-item interactions. To address this problem, in this paper we propose a novel recommendation model, i.e., adversarial neural network with multiple generators, to generate users from multiple perspectives of items’ attributes. Namely, the generated users are represented by attribute-level features. As both users and items are attribute-level representation, we can implicitly obtain user-item attribute-level interaction information. In the light of this, we can recommend new item to users based on attribute-level similarity. Extensive experimental results on two item cold-start scenarios, movie and goods recommendation, verify the effectiveness of our proposed model as compared to state-of-the-art baselines.
FRAMEWORK
![@2`OXBG92H4PR]]25@J[8GC.png](https://static.wixstatic.com/media/027513_e92ce66c3f4e43ab90e0451011fef094~mv2.png/v1/fill/w_606,h_314,al_c,q_85,usm_0.66_1.00_0.01,enc_avif,quality_auto/%402%60OXBG92H4PR%5D%5D25%40J%5B8GC.png)
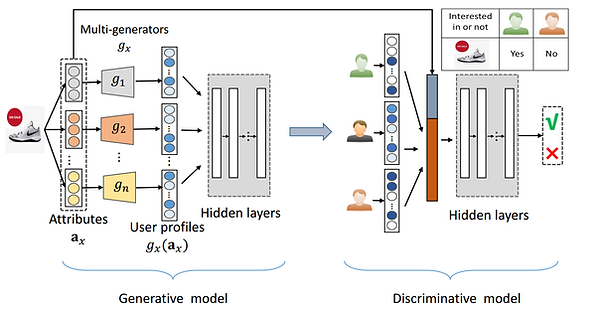